|

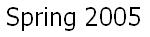

The topics studied in this course will include:
- Image statistics, image representations, and texture models
- Color Vision
- Graphical models, Bayesian methods
- Markov Random Fields, applications to low-level vision
- Approximate inference methods
- Statistical classifiers
- Clustering & Segmentation
- Object recognition
- Tracking and Density Propagation
- Visual Surveillance and Activity Monitoring

Lecture |
Date |
Description |
Readings |
Assignments |
Materials |
1 |
2/1 |
Course Introduction
Cameras and Lenses
|
Req: FP 1.1, 2.1, 2.2, 2.3, 3.1, 3.2
|
PS0 out |
Lecture 1
Lecture 1 (6 slides/page) |
2 |
2/3 |
Image Filtering
|
Req: FP 7.1 - 7.6
|
|
Lecture 2
Lecture 2 (6 slides/page)
Matlab Code Tutorial |
3 |
2/8 |
Image Representations: Pyramids |
Req: FP 7.7, 9.2 Handout 1
|
|
Handout 1
Lecture 3
Lecture 3 (6 slides/page) |
4 |
2/10 |
Image Statistics |
Handout 2 |
PS0 due
|
Handout 2
Lecture 4
Lecture 4 (6 slides/page) |
5 |
2/15 |
Texture |
Req: FP 9.1, 9.3, 9.4 |
PS1 out |
Lecture 5
Lecture 5 (6 slides/page)
HeegerBergen Texture Synthesis Code |
6 |
2/17 |
Color |
Req: FP 6.1-6.4 |
|
Lecture 6
Lecture 6 (6 slides/page) |
|
2/22 |
No class (Presidents Day - Monday class to be held) |
|
|
7 |
2/24 |
Guest Lecture:
Context in Vision |
|
PS1 due |
Lecture 7
Lecture 7 (4 slides/page) |
8 |
3/1 |
Local Features for Tracking |
Req: Lowe |
PS2 out |
Handouts 3 - 4
Lecture 8
Lecture 8 (6 slides/page) |
9 |
3/3 |
Features and Geometry |
Req: Mikolajczyk and Schmid; Belongie et al |
|
Handouts 5 - 6
Lecture 9
Lecture 9 - Shape Context
Lecture 9 (6 slides/page)
Lecture 9 - Shape Context (6 slides/page) |
10 |
3/8 |
Model Based Recognition |
Req: FP 18.1-18.5 |
|
Lecture 10
Lecture 10 (6 slides/page) |
11 |
3/10 |
Bayesian Analysis |
Req: Ch. 1 of Bishop (handout in class) |
PS2 due (Mar. 14 5pm)
|
Lecture 11
Lecture 11 (6 slides/page) |
12 |
3/15 |
Markov Random Fields
Belief Propagation |
Req: Jordan and Weiss Opt. Murphy |
EX1 out |
Handout 7
Lecture 12
Lecture 12 (6 slides/page) |
13 |
3/17 |
More on Graphical Models |
|
EX1 due |
Lecture 13
Lecture 13 (6 slides/page) |
|
3/22-3/24 |
Spring Break (NO LECTURE) |
14 |
3/29 |
More on Graphical Models |
|
|
Handout 9
Lecture 14
Lecture 14 (6 slides/page) |
15 |
3/31 |
Medical Applications of Computer Vision |
|
Project proposal due
PS3 out |
Lecture 15 |
16 |
4/5 |
Face Detection and Recognition I |
Req: FP 22.1-22.3
|
|
Lecture 16
Lecture 16 (6 slides/page) |
17 |
4/7 |
Face Detection and Recognition II |
|
|
Handout 10-12
Lecture 17
Lecture 17 (6 slides/page) |
18 |
4/12 |
Object Recognition |
|
PS3 due |
Lecture 18
Lecture 18 (6 slides/page) |
19 |
4/14 |
Segmentation and Clustering |
Req: FP 14, 15.1-15.2, Comaniciu and Meer |
PS4 out
|
Handout 15
Lecture 19
Lecture 19 (6 slides/page) |
|
4/19 |
No class (Patriot's Day Holiday) |
|
|
20 |
4/21 |
Computer Vision for Interactive Computer Graphics |
|
|
Handout 16
Lecture 20
Lecture 20 Part2
Lecture 20 (6 slides/page)
Lecture 20 Part2(6 slides/page) |
21 |
4/26 |
Prof. Daniel Huttenlocher's Talk |
|
|
|
22 |
4/28 |
Tracking I |
Req: FP 17
|
PS4 due |
Lecture 21
Lecture 21 (6 slides/page) |
23 |
5/3 |
Particle Filters, Tracking humans, |
Req: FP Extra Chapter
|
EX2 out |
Handout 17
Lecture 22
Lecture 22-KTH
Lecture 22 (6 slides/page)
Lecture 22-KTH (6 slides/page) |
24 |
5/5 |
Tracking Humans How to Write Papers and Give Talks |
|
EX2 due |
Lecture 23
Lecture 23 (6 slides/page) |
25 |
5/10 |
Applications: Motion Microscopy and Separating Shading from Paint |
|
|
Lecture 24a
Lecture 24b
Lecture 24a (6 slides/page)
Lecture 24b (6 slides/page)
|
26 |
5/12 |
Project Presentations
|
|
Project due |
|

- E. H. Adelson, E. P. Simoncelli, and W. T. Freeman, Pyramids and Multiscale Representations. In Representations of Vision , pp. 3-16, 1991.
- S. Lyu and H. Farid , How Realistic is Photorealistic? . In IEEE Transactions on Signal Processing , 53(2):845-850, 2005.
- D. G. Lowe, Distinctive Image Features from Scale-Invariant Keypoints.
In International Journal of Computer Vision , 2004.
- Allan Jepson , Local Feature Tutorial .
- K. Mikolajczyk and C. Schmid, A performance evaluation of local descriptors.
In IEEE Conference on Computer Vision and Pattern Recognition, pp. 257-263, 2003.
- S. Belongie, J. Malik and J. Puzicha, Shape Matching and Object Recognition Using Shape Contexts.
In IEEE Transactions on Pattern Analysis and Machine Intelligence, Volume 24 , Issue 4 (April 2002), pp. 509 - 522.
- M.I. Jordan and Y. Weiss, Probabilistic inference in graphical models.
In Arbib, M. (ed): Handbook of Neural Networks and Brain Theory. 2nd edition. MIT Press, 2002.
- Kevin Murphy, A Brief Introduction to Graphical Models and Bayesian Networks 1998.
- Matlab MRF example codes .
- Baback Moghaddam and Alex Pentland, Probabilistic Visual Learning for Object Representation .
In Early Visual Learning, Oxford University Press, 1996.
- B. Moghaddam and M-H. Yang, Gender Classification with Support Vector Machines.
In Proceedings of the 4th IEEE Int'll Conf. on Face and Gesture Recognition, 2000.
- B. Moghaddam, T. Jebara and A. Pentland, Bayesian Face Recognition.
In Pattern Recognitionn, Vol. 33, No. 11, pps. 1771-1782, November, 2000.
- Bernhard Scholkopf, Statistical Learning and Kernel Methods.
In Microsoft Research Technical Report, 2000.
- M. Weber, M. Welling and P. Perona, Unsupervised Learning of Models for Recognition.
In ECCV, 2000.
- D. Comaniciu and P.Meer, Mean Shift: A Robust Approach Toward Feature Space Analysis.
EEE Transactions on Pattern Analysis and Machine Intelligence, 2002.
- W. Freeman, How to write a conference paper..2002.
- D. Forsyth and J. Ponce, Computer Vision: A Modern Approach, Extra Chapter.
Prentice Hall, 2002.
home | syllabus | problem
sets and exams | grading and
requirements | internet resources
This site is maintained by Xiaoxu Ma.
|